
The benefits of predictive maintenance
As part of our increasingly connected world, companies are now able to use technology to manage their assets more efficiently, which has led to the rise of predictive maintenance (PdM).
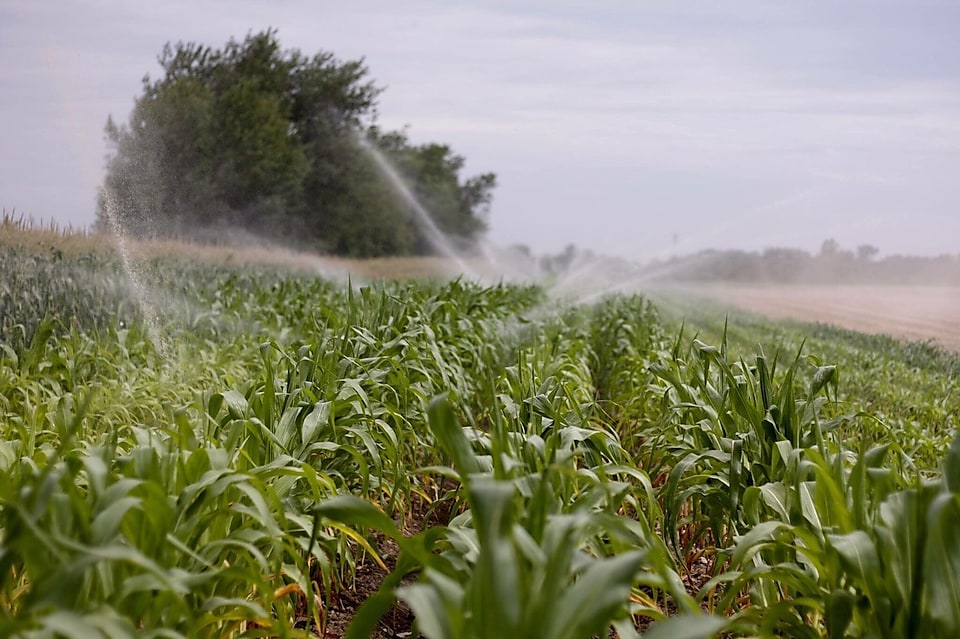
How predictive maintenance can save time, money - and even lives
This article will look at the latest developments in the field, covering:
- How can predictive maintenance help protect assets?
- Are there cost savings to be made?
- Will the nature of sensing change?
- What are the applications of predictive maintenance?
- Next steps for predictive maintenance
- Is AI a technology that can help?
How can predictive maintenance help protect assets?
Predictive maintenance (PdM) – which is aimed at reducing the likelihood of failures and, as a result, avoid costly downtime and lower maintenance costs – comes to the fore when companies address asset management. PdM can be implemented by monitoring the condition and performance of equipment during its normal operation, and using sensor technology and computerised maintenance management system (CMMS) software.
Alongside the sensors, PdM software can access a number of different data sources in real time, which can then predict asset failure or quality issues. These solutions use predictive analytics to detect any anomalies and failure patterns, determining where any problems or failures are likely to occur.
Dirk Claessens, IBM managing director for its Royal Dutch Shell account, explained how this technology all comes together in real-world applications, saying: “The objective is an increase in reliability of the overall asset or part of the asset.” “The predictive part is not just taking data from the automation piece, but a whole host of data: it could be maintenance logs, weather data, production forecasts and so on.”
“The objective is an increase in reliability of the overall asset or part of the asset.”
“It’s all about reliability. Depending on where you come from – whether you are an OEM manufacturer equipment supplier or an owner operator – you will have a different viewpoint.”
Claessens also explained how owner operators tend to be more interested in reliability in the overall operation, with the result that they’re more interested in how the maintenance service orders affect the overall production performance. OEMs, on the other hand, are more interested in becoming more efficient and effective in the machine itself. This is a more limited scope, in order to provide a better service to their clients and isn’t necessarily tightly integrated with the overall planned performance.
Are there cost savings to be made?
The cost of that increased reliability is not to be discounted, either. A McKinsey Global Institute report, The Internet of Things: Mapping the Value Beyond the Hype, suggested that manufacturers’ savings from predictive maintenance could globally total between $240 and $630bn by 2025.
That’s a lot of money, which makes academic research into predictive maintenance a worthwhile investment.
Professor Julie McCann, from London’s Imperial College, is one such academic. She and her team have been studying how PdM systems are being adopted in industry – and how they can be improved: “Sensing has been in industry longer than trendy terms such as IoT.”
The vibration sensors that have previously been used, stretching back to the 1950s, in some cases, are wire-based sensors and quite expensive, which meant that they were used sparingly.
McCann added: “What we’re seeing at the moment is the lower cost price per sensor and the delivery mechanism has become wireless – which means you can place sensors in more awkward and difficult-to-get-to areas. Technology is now more fitting to be able to place sensors around and monitor these kinds of systems. And the cost benefit now is much more favourable to ship these systems with the sensors in them.”
“What we’re seeing at the moment is the lower cost price per sensor and the delivery mechanism has become wireless.”
Indeed, an analysis of the sensor market by IC Insights, a US-based semiconductor market research company shows that average selling prices (ASPs) on sensors have fallen from around $0.66 in 2010 to $0.40 in 2015. The report also forecasts that sales of microelectromechanical system (MEMS)-based sensors will rise by 5.5% to $6.1 billion in 2020.
Will the nature of sensing change?
Sensors aren’t the only game in town, however, as Professor McCann (Imperial College, London) explained: “Our research is looking at alternatives, because placing physical sensors is expensive. You have to place them, they only measure the area in which they're placed and not the whole machine, and they also have to maintain them and ship all that data back.
“We’re looking at an alternative, which is throwing radio frequency signals through [machines] from afar. It's the equivalent of flooding it with your normal, everyday wifi network, reading how the network behaves, and then analysing how the machine behaves because of that. We call it CogniSense, or sensing without sensors, because you’re not placing a sensor on the actual device itself.”
CogniSense is a remote condition-monitoring radio- frequency device and has been devised and patented by leading researchers from Imperial College London . This technology can obtain more data than could ever be achieved with point-based sensors.
"We call it CogniSense, or sensing without sensors, because you’re not placing a sensor on the actual device itself.”
The thinking behind CogniSense is that any physical movement produces a recognisable footprint on a Radio-Frequency (RF) signal interacting with it. If a system can therefore transmit a radio signal and detect the response, a signal corresponding to the motional speeds of the components can be captured and analysed. This can point to the condition of the machine.
Correctly calibrated, Imperial’s researchers think that CogniSense could be capable of determining the overall condition of a machine at a system-level. And not only that: at a component-level, different internal rotational speeds (e.g. bearings) that affect the system integrity could also be monitored.
Laboratory experiments so far have involved monitoring home appliances such as ventilation fans. The measured speeds correspond with those captured by a laser tachometer with 100% accuracy. This has been achieved from a distance of several meters, and even through walls.
The aim of this technology is to reduce machine downtime by providing a single low-cost device to monitor numerous non-instrumented machines, or monitoring machines that might already be instrumented, but aren’t connected to a digital network able to transmit the data.
“The other advantage is that when you put a sensor on a device, it's only measuring in its local area, pinpointing the area it’s attached to or focused on: it's not the whole machine,: McCann added. “What systems like CogniSense are looking at is more volumetric: it's the whole system, and the interactions between the components and the whole system that it’s measuring, so it’s able to understand the coupling in the system and all those kinds of things. It’s getting you much more information, as well as being more convenient and super-low in cost.”
What are the applications of predictive maintenance?
With developments such as this, PdM is already becoming more subtle than just predicting machinery failures and helping to plan maintenance. IBM’s Claessens told us: “People have moved beyond simply ‘This machine is going to break down in the next 48 hours’: it’s about predicting service degradation. So there’s going to be a service degradation in a part of the equipment and these are the actions you can take to prevent it from happening. One of the outcomes could be the breakdown of the equipment, but it could be something else: it could be a change in the operation parameters or so on.”
While manufacturing production lines might be the most obvious application of PdM technology, it’s also becoming widely adopted in other industries.
“People have moved beyond simply ‘This machine is going to break down in the next 48 hours’: it’s about predicting service degradation."
Professor Ross McAree, head of the School of Mechanical and Mining Engineering at the University of Queensland, explained how the mining industry is also seeing the benefits of predictive maintenance.
He explained how PdM is currently being used in a wide range of applications throughout the mining industry, helping the efficiency of numerous operations. “Rotating machinery such as pumps are routinely instrumented and measurements taken to detect emergent faults, such as bearing failure. Large fixed plant, especially crushers and grinders, are critical to production and are instrumented to make predictions of maintenance. For at least 10 years most Australian draglines have been fitted with ‘duty monitors’ that predict weld failures on boom, using a mix of fatigue models and strain measurement. The large diesel engines used to power trucks and excavators are also heavily instrumented and measurements used to identify emergent failures, while conveyor lines are instrumented to detect bearing failures using vibration signatures.”
Safety is another important use case for predictive maintenance in the mining industry. Claessens said: “We did a study of the coal-mining industry in China, where 3,000 people are killed every year and 80% of these deaths were caused by equipment failure. So there’s a clear connection to a safety use case for predictive maintenance.”
The agricultural industry is another where machinery such as harvesters is starting to incorporate sensors that monitor key areas of the machine. The sensor data is transmitted via a mobile phone network and cloud computing to a central maintenance service, which can analyse the data to detect irregularities and predict problems in advance.
Agriculture is becoming increasingly mechanised. Machines such as harvesters and tractors are now commonplace, especially on large farms; automated irrigation systems are vital to operation in many parts of the world; and even robots that can kill weeds growing among crops, top up fertiliser levels and pick crops are currently being developed and tested. With all these becoming increasingly important to modern farming operations, the need for PdM systems will rise.
“We did a study of the coal-mining industry in China, where 3,000 people are killed every year and 80% of these deaths were caused by equipment failure."
This isn’t just about increasing the efficiency of machinery, though: PdM technology could play a vital part in the future of the world’s population. As McCann said: “Farming and agriculture also use these systems, not just for the machinery, but also for making the most of the land without having to put resources into it.
“That’s important because we’re going to have to feed a lot more people by 2050 and we don’t have enough acres of arable land on the planet to do that, so we’re going to have to become a lot more efficient with the land. These kinds of systems are helping with that as well.”
Next steps for predictive maintenance
Some industries are still in the early stages of the technology’s adoption, however. Claessens said: “From an owner operator perspective, we’re in the proof of concept or pilot stage. I haven’t seen industrial clients go for a full-blast, end-to-end implementation of predictive analytics around maintenance. People are playing around with the technology.
“Where you do see programmes that are ongoing and end-to-end is in OEMs. If you look at a company such as Kone, the elevator company, that’s a good example. They have one million assets around the world and they’re retrofitting sensors and data capture equipment. From their perspective, they have a strong driver, from a business standpoint, to implement this technology end-to-end for two reasons. Number one: they want to keep control of the maintenance and spare parts revenue stream, because it’s typically where they earn their margin. And at the same time they want to explore new revenue streams, so can they start providing services to their clients, while they’re getting control of all the data.”
Mining has been further ahead of the curve, though. “Approximately 40% of the costs of mining are associated with management of the assets and so the need for predictive maintenance technology has been long understood and the benefit is significant,” McAree explained.
And as it’s further down the road, mining is also looking at more advanced systems. “What we are seeing a lot of at present is people talking about using machine learning methods based on big data for fault prediction and there is some enthusiasm for this for a couple of reasons,” McAree added. “First, machine learning algorithms give a fresh perspective to a long-term need. Second, there is a lot of data collected from which little value is derived – its collection is a cost without a clear benefit at present, so machine learning methods offer the prospect of a benefit. These efforts are still in their infancy and, while there have been some wins, there is still a long way to go before we see a step change in practice.”
“What we are seeing a lot of at present is people talking about using machine learning methods based on big data for fault prediction”
Is AI a technology that can help?
Claessens also sees artificial intelligence (AI) as the next stage in PdM’s evolution and expects that the next generation of analytics solutions around asset reliability will be based on the emerging cognitive AI.
He said: “As an example, you get a signal from data – sensor data or any suite of data that allows you to build robust models around asset reliability – so the sensor will tell you that in this part of the equipment there’s a service degradation likely to happen in the next three days. The data will be picked up by an AI module that would read into anything that’s available, both within the owner operator’s data suite or outside it, to identify a probable cause of this service degradation and give you a recommendation for the actions to fix it.
“Service supervisors can look at this and they’ve got a list of service orders to schedule, this is a profile of the service technician that they need, and these are the spare parts and tools they’ll need to fix it. So you’ll always have a fix in the first instance.”
Initial applications of machine learning point to numerous benefits for companies employing it for PdM. Better availability and reliability of assets is certainly one that companies will discover, as is reduced capital expenditure (improved asset availability means fewer assets are needed). There should also be lower operational expenses, thanks to fewer maintenance hours and replacement parts. Estimates from McKinsey (in its 2017 report Smartening up with Artificial Intelligence (AI) -What’s in it for Germany and its Industrial Sector?) suggest that AI-enhanced predictive maintenance can lead to savings of 20% or more on maintenance costs for key systems.
“Initial applications of machine learning point to numerous benefits for companies employing it for PdM.”
McCann agrees that AI will have an important role to play: “To be able to hone in on a single component, you need to be able to understand what that component is, how to identify it in the big signal coming in and pinpoint it within all the signal mess. To be able to do that, we need to be able to use machine learning.
“So in the future, we can imagine using AI if the machine wants to self-recalibrate or have a notion of reconfiguration that it does itself, based on its own results coming in.”
Solutions such as predictive maintenance will only become more important to the smooth running of numerous industries, as the amount of technology used in industry increases further. These industries will also receive a wide range of benefits, from lower costs to increased safety.
And with the introduction of AI, predictive maintenance is an area that can only help industry become more efficient and productive. As businesses evolve, so does the world of lubricants. A machine that can predict faults also has the potential to predict lubrication contaminations, thus helping reduce machine downtime and increasing the lifetime of your products.
Featured content
Distributor Locator
Where to buy Shell oils and lubricants.
Sector Expertise
Discover Shell’s expertise in providing our customers the right products and the right advice across various sectors.